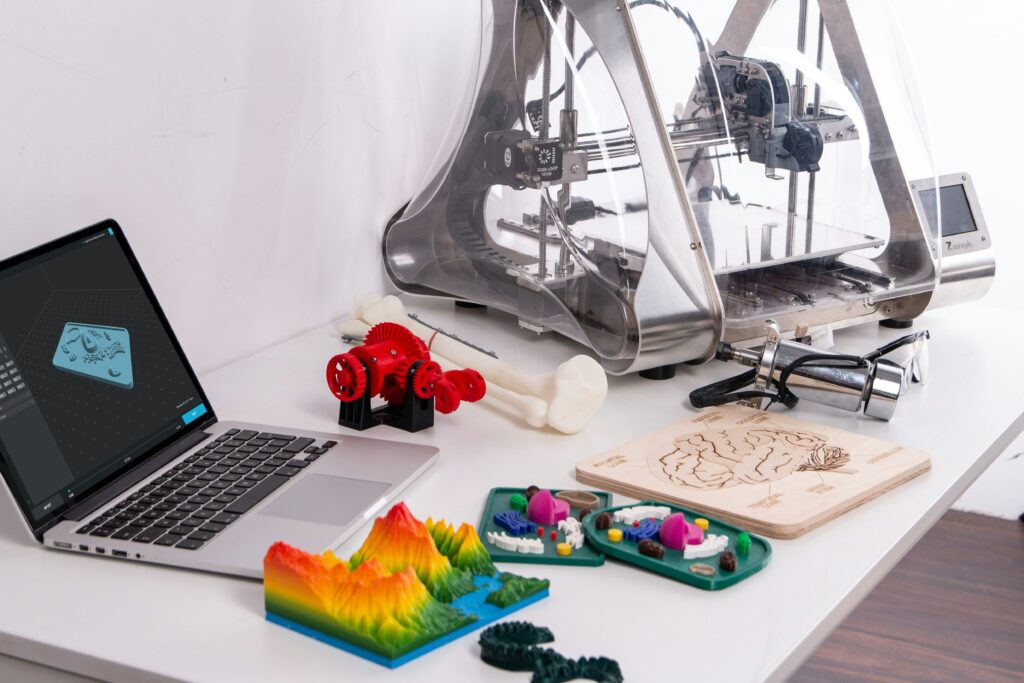
Artificial Intelligence (AI) and machine learning have significantly impacted various industries, with education being one of the sectors experiencing rapid change. In the realm of STEM (Science, Technology, Engineering, and Mathematics), machine learning is proving to be a game-changer. By integrating machine learning into STEM education, educators can enhance problem-solving skills, provide personalized learning experiences, and inspire innovation. This blog post explores how machine learning is reshaping STEM education, offering students the tools they need to succeed in a technology-driven world.
Understanding Machine Learning in STEM Education
Machine learning involves training computer systems to learn from data, identify patterns, and make decisions with minimal human intervention. In the context of STEM education, machine learning enables the development of intelligent systems that can support and enrich the learning process. From interactive simulations to intelligent tutoring systems, AI-driven tools can adapt to each student’s learning pace, making STEM subjects more accessible and engaging.
A growing number of educational institutions are adopting machine learning solutions to transform traditional STEM learning environments. These technologies not only facilitate complex problem-solving exercises but also encourage critical thinking and analytical skills, which are crucial for students pursuing STEM careers.
For students who need additional support in their academic pursuits, options like to buy dissertation from PaperWriter can offer valuable guidance. Combining human expertise with AI-driven tools creates a comprehensive learning experience tailored to individual needs.
Enhancing Problem-Solving Skills with Machine Learning
One of the most significant benefits of integrating machine learning into STEM education is its ability to enhance students’ problem-solving skills. Here’s how AI-based learning tools help students become better problem solvers:
1. Adaptive Learning Systems
Machine learning-driven adaptive learning systems provide personalized educational experiences tailored to each student’s needs. These systems monitor students’ progress, identify areas of difficulty, and adapt the learning material accordingly. For example, if a student struggles with calculus problems, the system offers additional resources and exercises to help them understand the concept better.
By customizing the learning process, adaptive systems enable students to tackle complex problems at their own pace. This personalized approach ensures that students receive adequate support in areas they find challenging, thereby enhancing their overall problem-solving abilities.
Learn more about adaptive learning systems and their impact on education in this educational article.
2. Intelligent Tutoring Systems
Intelligent tutoring systems (ITS) leverage machine learning algorithms to simulate human tutoring. These systems provide real-time feedback, monitor students’ learning paths, and offer suggestions for improvement. In STEM education, ITS can help students practice problem-solving by offering hints and step-by-step guidance, promoting a deeper understanding of complex concepts.
For instance, an intelligent tutoring system in a physics class might help students work through difficult equations by offering hints when they make mistakes. By receiving immediate feedback, students can learn from their errors and develop critical problem-solving skills.
Additionally, these systems are available 24/7, allowing students to seek help outside classroom hours, which is particularly useful when students are engaged in projects like buying a dissertation paper for in-depth research.
3. Simulation-Based Learning
Simulation-based learning is another area where machine learning plays a crucial role in enhancing STEM education. By using AI-driven simulations, students can engage in virtual experiments and explore real-world scenarios in a controlled environment. This hands-on approach helps them apply theoretical knowledge to practical problems, fostering innovative thinking.
For example, a machine learning-based chemistry simulation can allow students to experiment with different chemical reactions without the risks involved in a physical lab setting. By exploring different outcomes and learning from mistakes, students enhance their problem-solving abilities, gaining confidence in their understanding of scientific concepts.
For more insight into simulation-based learning in STEM, check out this research article.
Real-World Applications of Machine Learning in STEM
Machine learning is not only transforming classroom experiences but also preparing students for real-world applications. By introducing students to AI-based problem-solving tools, educators provide them with skills applicable in various STEM fields, from data science to engineering.
For students pursuing advanced degrees, the need to solve complex problems becomes even more prominent. In such cases, they may consider resources like buying a dissertation online to guide their research. Machine learning technologies also assist in this process, as AI-powered tools can help students find relevant academic papers, analyze data, and build models for their research projects.
Integrating Machine Learning into the Curriculum
While the benefits of machine learning in STEM education are evident, the key to success lies in its seamless integration into the curriculum. Educators should focus on incorporating AI tools into problem-solving activities rather than using them as standalone applications. This approach ensures that students develop a holistic understanding of STEM subjects while honing their analytical and critical thinking skills.
Collaborative Learning Environments
Machine learning can also foster collaborative learning environments where students work together on problem-solving tasks. AI-based platforms can facilitate group projects, allowing students to share insights and tackle challenges collectively. This collaborative approach enhances students’ ability to think critically and creatively, preparing them for future STEM careers.
Explore how AI is shaping collaborative learning in this article.
Challenges and Ethical Considerations
While machine learning offers numerous benefits in STEM education, it is essential to consider the challenges and ethical implications associated with its use. Data privacy, algorithmic bias, and the potential for over-reliance on AI tools are some concerns that educators must address when integrating machine learning into their teaching methods.
Ensuring that students use AI responsibly, especially when considering options like buying a dissertation online, is crucial to fostering a balanced educational environment. Educators must emphasize the importance of ethical practices, critical thinking, and human oversight in the learning process.
Conclusion
Machine learning is transforming STEM education by enhancing problem-solving skills, providing personalized learning experiences, and preparing students for real-world challenges. AI-driven tools, such as adaptive learning systems, intelligent tutoring, and simulation-based learning, are making STEM subjects more accessible and engaging for students of all levels.
As educators continue to integrate machine learning into the STEM curriculum, it is vital to address the challenges and ethical considerations associated with AI use in education. By fostering a responsible and balanced approach, students can harness the power of machine learning to become proficient problem solvers, critical thinkers, and innovative professionals.
For students seeking additional support, options like buying a dissertation paper offer further resources to complement their learning journey. However, the true value of education lies in the blend of human guidance and AI-driven tools that together create a rich, diverse learning experience.